ISO 17184:2014 pdf download.Soil quality — Determination of carbon and nitrogen by near-infrared spectrometry (NIRS)
5 Procedure
5.1 Preparation of samples Soil samples shall be prepared the same way as soils used for the instrument calibration. Any difference in sample preparation may influence the measurement. Sample preparation shall ensure a good homogenization of the sample. Sample preparation according to ISO 11464, particle size < 2 mm, is generally used. Air-dried or oven- dried samples can be used for analysis. The method is not suitable for samples with water content higher than 10 %. Oven drying is recommended for samples with high specific surface area that are susceptible to changes in water content due to fluctuations in air humidity.
5.2 Instrument calibration
5.2.1 General A suitable set of uniformly prepared soil samples is measured by NIR spectroscopy. The spectra and the results of the content of carbon and/or nitrogen determined by a reference method are used for calculation of the calibration model. Calibration should include enough samples to cover most of the possible spectral variability encountered during routine analysis and to predict the composition of unknown samples accurately.
The calibration sample set shall be selected to gain an evenly distributed coverage of the property range. The NIR spectra represent cumulative information about the chemical and physical properties of a sample. Influence of physical properties of a sample (e.g. particle size), is reduced by mathematical corrections as derivatives, standard normal variate (SNV), multiplicative scatter corrections (MSC), etc. There are several possible ways for development of calibration equations and no specific procedure can be given. The choice shall aim at minimising the calibration error.
The methods most frequently applied in the development of calibration equations are: PCR (principal component regression), PLS (partial least square regression), LWR (locally weighted regression), SMLR (stepwise multiple linear regression) and ANN (artificial neural network regression). Among these methods, only ANN methods can give calibration for the whole concentration range for carbon and nitrogen in soils but ANN methods only apply with more than 500 calibration samples. For other statistical methods, splitting of the concentration range into two calibrations was found to be the best solution. Removal of outliers from the calibration set usually reduces the robustness of the calibration and should be avoided. For samples from different locations and soil types, a minimum of 60 to 100 calibration samples is required. A smaller number of calibration samples can be used for sample sets with lower variability such as samples from a defined location.
NOTE 1 Transformations of the reference measurements or the spectra using e.g. log or square root transformed reference measurements may help to reduce the calibration error.
NOTE 2 It is possible that calibrations developed on a certain instrument may not always be transferred directly to an identical instrument. It may be necessary to perform bias and slope adjustments to calibration equations.
In many cases it is necessary to standardize the two instruments against each other before calibration equations can be transferred. Standardization procedures can be used to transfer calibrations between instruments of different types provided that samples are measured the same way and that the spectral region is identical. NOTE 3 If the reference method is unbiased and a good linear calibration model is achieved, increasing number of calibration samples averages out errors in the reference method. Therefore, the lack of repeatability in the reference method can be compensated for by using high number of calibrating samples.
5.2.2 Validation of the calibration model
5.2.2.1 General
There are two main methods for validation of the calibration model:
— cross (internal) validation (see 5.2.2.2), and
— external validation (see 5.2.2.3).
Cross validation (see 5.2.2.2) is used to determine the number of factors used for PLS by determining a minimum RMSECV (root mean square error of cross validation), and when not enough samples are available for external validation. The number of factors shall be as small as possible to avoid over fitting of the calibration model. An external validation (see 5.2.2.3) shall be used to determine the calibration error since cross validations tend to underestimate the calibration error.ISO 17184 pdf download.ISO 17184 pdf download
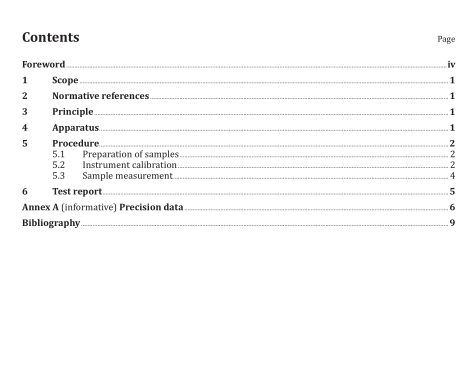